Modeling and Energy Management Strategy in Energetic Macroscopic Representation for a Fuel Cell Hybrid Electric Vehicle
전체 글
수치
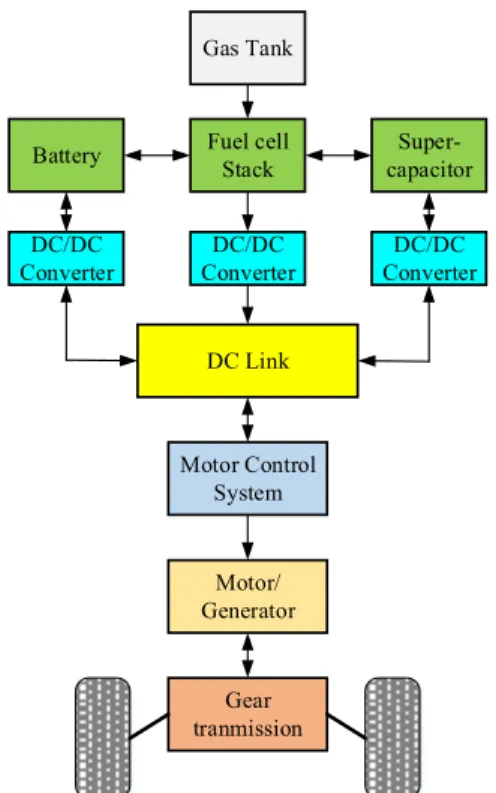
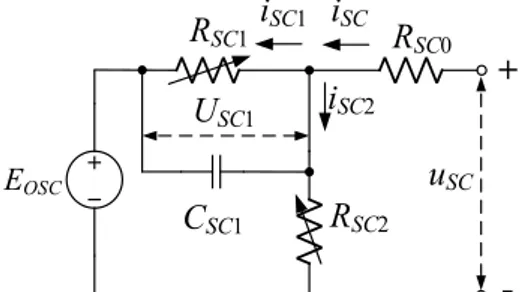
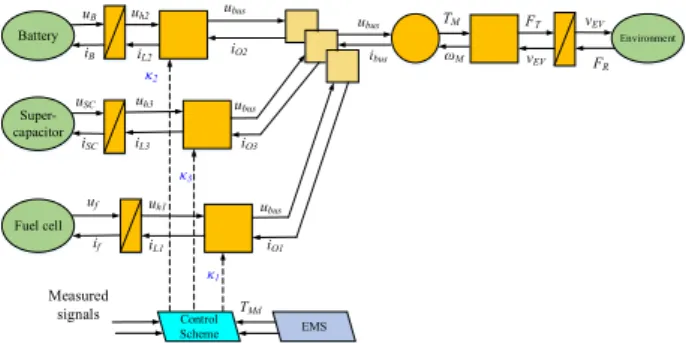
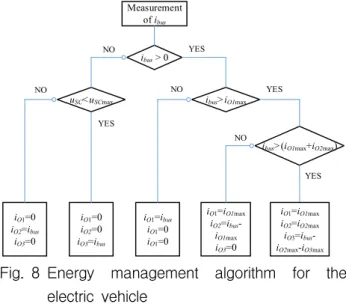
관련 문서
The construction of Electric motor propulsion system are presumed arrangement of boat and compared The engine fuel of cost with battery charge of cost .The Model
If this spring should happen to be part of a larger elastic system, it will always contribute the energy (2.3) to the total stored energy of the system
In this paper, we developed a data management system for shipboard machinery equipment and the monitoring application for the verification of the utility, and we
Regional Innovation Center for the Hydrogen Fuel-Cell and Solar
Basic construction of axial-lead tubular plastic-film dielectric capacitors Floyd 책 383쪽 그림 9.12. Polyester-film capacitors: (a) axial lead; (b) radial lead (Courtesy
The change in the internal energy of a closed thermodynamic system is equal to the sum em is equal to the sum of the amount of heat energy supplied to the system and the work.
The change in the internal energy of a closed thermodynamic system is equal to the sum em is equal to the sum of the amount of heat energy supplied to the system and the work.
This study focuses on the energy-related legal system of Australia and examines (1) the general regulatory framework of energy industries, (2) the management and oversight